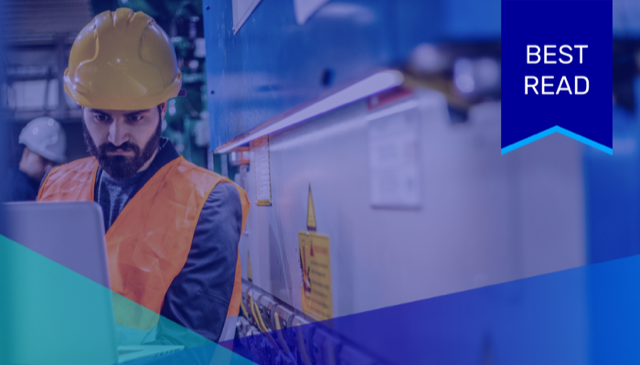
Every manufacturer wants to improve overall equipment effectiveness (OEE) and associated metrics, but without real visibility into machine health, achieving world-class OEE is impossible. In this article we outline how a predictive approach to maintenance can lead to significant improvements in a manufacturing operation’s OEE by maximizing machine availability and performance, as well as output quality.
This article was originally published on October 27, 2020.
As emerging technologies continue to transform manufacturing, traditional approaches to machine maintenance are no longer as effective. Maintenance and reliability teams utilizing a reactive approach to addressing equipment issues and breakdowns don’t have any real insights into machine health. As a result, they can’t make meaningful improvements to OEE.
In the increasingly competitive world of manufacturing, this is a major problem.
What Is OEE?
As the premier metric for measuring a manufacturing operation’s productivity, OEE considers equipment availability, performance, and quality of output to assign a numerical value to the overall effectiveness of a machine or production line. Essentially, that numerical value represents a machine’s actual productivity in relation to its full potential over the course of planned operations. It’s expressed as a percentage.
A low OEE value means that a production line or individual piece of equipment is severely underperforming, whereas an OEE of, say, 100% indicates that an operation is producing only the highest-quality parts, as fast as possible, for the entire duration of planned production time.
Every manufacturer wants to improve OEE and its associated metrics, but achieving world-class OEE is virtually impossible without real visibility into machine health.
Time for a Change
The typical manufacturer allocates approximately 40% of total operating expenditure to maintenance — a substantial amount for any company. Yet when maintenance and reliability teams operate within a traditional reactive or preventive framework for identifying and prioritizing equipment repair, that investment is often insufficient.
Factory walk-throughs, random equipment checks, and annual shutdowns don’t just consume valuable time; they’re also prone to human error and leave opportunities for machine malfunctions to cause unplanned downtime. Furthermore, the data generated by these methods generally isn’t useful for manufacturers trying to forecast future risk and production output.
As a result, manufacturers employing conventional maintenance practices experience more unplanned downtime, lower-quality output, and more maintenance-related costs throughout the year. Eventually, these negative outcomes add up, putting a manufacturing operation at a severe disadvantage in relation to more forward-thinking competitors.
So what’s the solution?
How to Improve OEE
Given the increasingly complex nature of modern factory equipment, manufacturers can’t afford to not continuously monitor machine health. Real-time insight into equipment performance generated by sensors mounted onto or embedded within individual machines allows teams to perform condition-based and even predictive maintenance. The advantages of this approach over outdated maintenance and monitoring techniques are clear and substantial.
By using vibration analysis and similar data-collection methods in conjunction with advanced analytics, factory operators can anticipate a machine failure before it happens. When a bearing starts to show signs of deterioration, for example, teams utilizing a condition-based maintenance approach can replace the part before it negatively impacts a machine’s effectiveness.
Not only does condition-based maintenance allow manufacturers to reduce machine downtime and avoid temporary unplanned outages (as well as potentially catastrophic equipment failure), but it also allows them to accurately forecast future risk of failure based on a machine’s current condition. By extension, they’re able to more confidently set production targets and plan future maintenance operations around production goals.
To better understand how a predictive approach to maintenance can lead to significant improvements in a manufacturing operation’s OEE, let’s take a deeper dive into the metric’s three primary components.
1. Machine Availability
In high-level sports, it’s often said that the best ability an athlete can possess is availability. The same is true of machines in the world of manufacturing. A piece of equipment that’s not functioning at capacity (or at all) when you need it to be operating at 100% is a financial drain on your business. Unscheduled downtime as a result of machine failure is one of the largest obstacles manufacturers face when it comes to improving OEE. A reactive approach to maintenance virtually guarantees that unexpected equipment failure will be a persistent problem.
Manufacturers that rely on a preventive maintenance approach, with scheduled downtime to address equipment issues that may or may not impact OEE, face similar inefficiencies. During a plant shutdown or other scheduled maintenance periods, mechanics and technicians are typically presented with a task checklist and a target completion rate. In order to meet this target within the time allotted for maintenance, they’ll often mark unfinished repairs as complete. This is why a facility with a preventive maintenance checklist that is close to 100% complete can find itself operating at just 50% efficiency.
Moreover, even the best technicians are prone to human error. During scheduled downtime, these experts will often be tasked with inspecting equipment that doesn’t need repair, resulting in further time and cost inefficiencies.
2. Machine Performance
In order to meet the demands of modern consumers, manufacturers must produce as much as possible, as fast as possible. The problem is that an outsize focus on production speed can actually lead to more instances of equipment failure, ultimately hindering productivity rather than improving it. Despite this reality, manufacturers must maintain certain performance standards if they hope to stay in operation.
Idling equipment and minor stoppages due to operational inefficiencies can become major problems if not addressed promptly. However, factories that rely on manual machine inspection processes often don’t know that a machine isn’t performing at full capacity until it fails completely. As a result, they suffer a loss of production that’s exacerbated by the need to divert human and capital resources to diagnostic and maintenance activities.
3. Output Quality
Large manufacturing operations have little room for error when it comes to production quality — they have to get it right the first time. If they don’t, they stand to lose potentially significant amounts of time and money and could suffer severe damage to their reputation as well.
Excess waste — scrap or output that can’t be sent to customers due to structural or cosmetic defects — often occurs when machines aren’t operating at optimal conditions. Whether this results from normal depreciation, overuse, or some other issue, it’s a loss for a manufacturer.
Each of these components can be improved manually over time, but if your goal is to achieve world-class OEE, reactive and preventive maintenance approaches won’t get you there — and they certainly won’t get you there ahead of your competitors. Instead, you’ll need a machine health monitoring solution that gives you real-time visibility into equipment status, condition, and performance.
But understanding the actual state of your machines with a machine health platform is only the first step toward improving OEE. Being able to use those insights to prioritize maintenance schedules and take direct, targeted action to reduce machine downtime, failures, and waste represents the next stage of facility sophistication.
A Holistic Approach to OEE
Within every manufacturing operation, there is a production and a maintenance unit, and it’s logical to assume that the two might work together to pursue common goals. Historically, however, that hasn’t been the case. In fact, their operational mandates have often put these two teams directly at odds with one another.
Maintenance is typically charged with maximizing OEE, while production teams’ objectives tend to shift based on ongoing revisions to quotas or product specifications. Both units are indispensable, but the emphasis on speed and the immense pressure to meet market demands mean production goals are usually prioritized over machine health and maintenance. Inevitably, this leads to losses in productivity and excessive spending on maintenance.
In the latter half of the 20th century, Japanese manufacturing leaders seeking to remedy this situation developed a methodology designed to create synergy between the two teams: Total Productive Maintenance (TPM). The focus of TPM is on reducing operational costs through the continual improvement of equipment effectiveness, and its creators established OEE as a critical metric in manufacturing.
While industry leaders have understood and implemented TPM’s principles for decades, relatively recent advances in machine learning and data analytics have allowed manufacturers to truly bridge the gap between maintenance and production — in a way that the creators of TPM could only imagine.
The Next Evolution of Manufacturing
TPM makes everyone responsible for OEE and calls for a holistic approach to production and maintenance. When manufacturers have access to real-time machine health data, such an approach becomes possible.
A machine health data platform gives factory operators insight into key equipment metrics (e.g., speed, vibration, heat levels), enabling them to decide whether a machine requires intervention with pinpoint accuracy. These capabilities allow operations to move from preventive maintenance to predictive maintenance, opening up countless opportunities to improve overall manufacturing productivity and efficiency.
With this data, for example, operators don’t just know whether they have the equipment necessary to meet production goals on schedule; they also know whether their machines can support faster or longer production runs. They can then adjust production line speeds or switch production lines altogether to sidestep equipment problems. Without machine health data, however, operators often find themselves gambling on the unknown.
When machine health metrics decline, a machine health platform will alert managers that maintenance is needed before issues arise. The technicians performing repairs then have access to a vast array of data that allow them to work more effectively and efficiently. On a macro level, machine health data can be combined with operational data, allowing manufacturing leaders to optimize facility design in a way that maximizes the OEE of individual machines. While it may seem counterintuitive, the reality is that a single optimized machine can actually cause downstream problems and reduce the efficiency of a production line if other equipment hasn’t been similarly optimized.
For example, consider a bottle-filling machine configured to fill 1,000 bottles every minute. A two-minute accumulation time between the filler and downstream labeler means that the labeler will be shut down for two minutes when label rolls need to be replaced. Suppose the filler is “optimized” to fill bottles at a clip that exceeds 1,000 units per minute but the label roll replacement process hasn’t also been improved. In that case, bottles will be filled faster than they can be labeled, resulting in excess waste.
Without a machine health platform, operators facing the above scenario might shut down the entire production line for equipment inspection and conclude that the problem is a faulty labeler. However, with the right data, an operator can avoid unplanned downtime and simply reduce the filler’s speed to reset the line and continue production, without having to spot-check each machine individually.
The benefits of a predictive maintenance approach paired with real-time machine health monitoring are both instantaneous and cumulative over time. Manufacturers that adopt such an approach will be able to continuously improve OEE, allowing them to set increasingly aggressive production goals and deadlines.
In the competitive world of 21st-century manufacturing, these operations will quickly become customer favorites, while those that persist with a reactive or preventive approach to maintenance will fall behind and eventually disappear. With early adopters of sophisticated machine health monitoring and data analytics solutions already separating themselves from their peers, the window of opportunity for latent adopters to close the gap is shrinking fast.
Want to talk more about pumping up your OEE? Reach out!