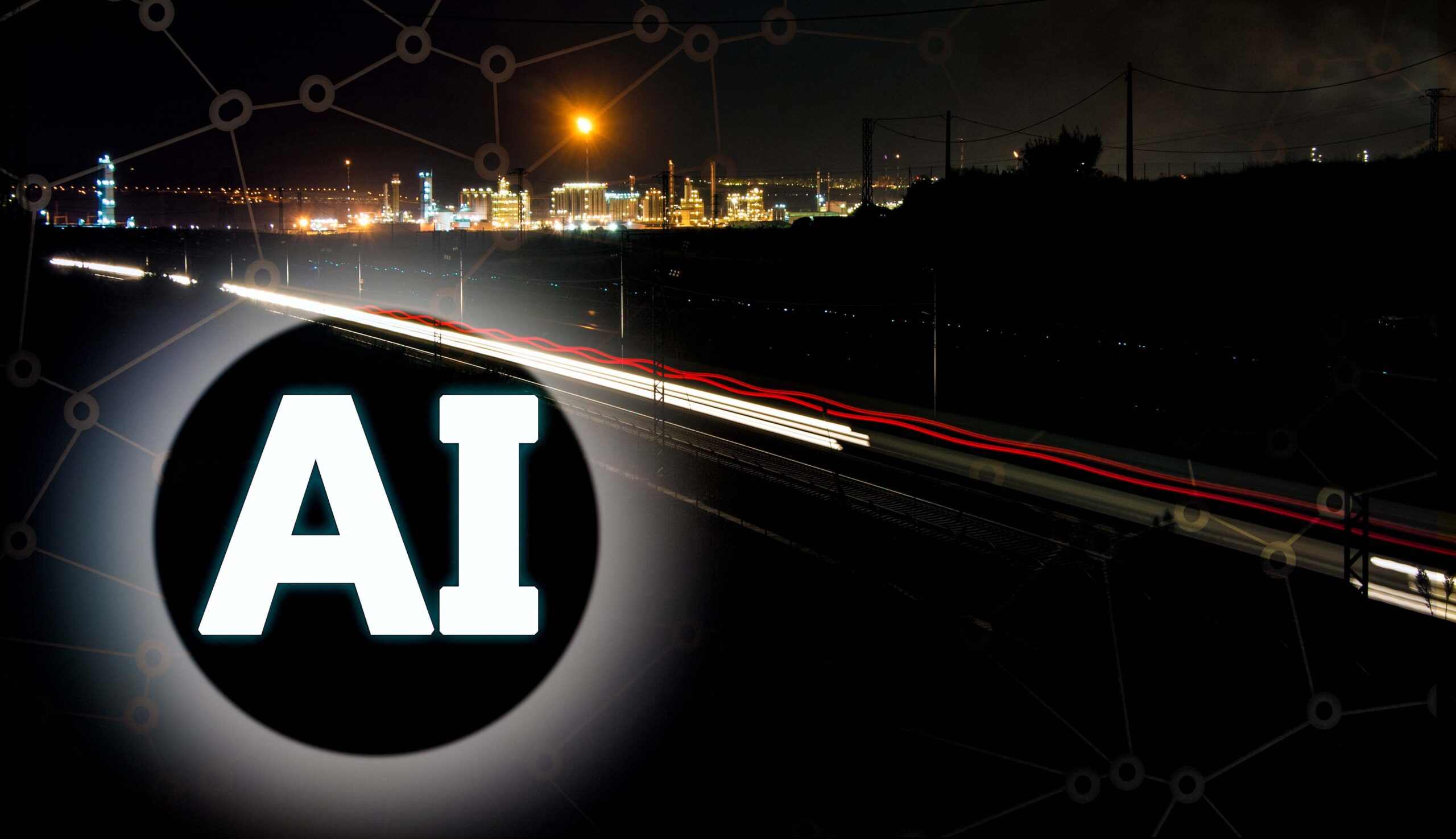
It’s a timeless manufacturing goal: producing more, higher-quality products at minimum cost. The “Smart Manufacturing” revolution is already enabling manufacturers to reach this goal more successfully than ever. And one of the main core technologies driving this new wave of innovation is Artificial Intelligence.
Data has become a highly valuable resource, and it’s cheaper than ever to capture and store. Today, more manufacturers than ever are leveraging that data to significantly improve their bottom line thanks to Artificial Intelligence – and particularly Machine Learning.
For many, this means greatly improving production efficiency and capacity, by eliminating the primary causes of production losses and other associated costs.
Of course, getting tangible business value out of Artificial Intelligence is often easier said than done. AI is a complex technology, with many different applications. How can manufacturers see through the “hype” and empty promises, to invest in Industrial AI that will truly give them a competitive edge?
The Groundbreaking Benefits of AI and Machine Learning for Manufacturing
The introduction of AI and Machine Learning to industry represents a sea change with many benefits that can result in advantages well beyond efficiency improvements, opening doors to new business opportunities.
Some of the direct benefits of Machine Learning in manufacturing include:
- Reducing common, painful process-driven losses such as yield, waste, quality and throughput
- Increased capacity by optimizing the production process
- Enabling growth and expansion of product lines at scale due to a more optimized process
- Cost reduction through Predictive Maintenance. PdM leads to less maintenance activity, which means lower labor costs and reduced inventory and materials wastage.
- Predicting Remaining Useful Life (RUL). Knowing more about the behavior of machines and equipment leads to creating conditions that improve performance while maintaining machine health. Predicting RUL does away with “unpleasant surprises” that cause unplanned downtime.
- Improved supply chain management through efficient inventory management and a well monitored and synchronized production flow.
- Improved Quality Control with actionable insights to constantly raise product quality.
- Improved Human-Robot collaboration improving employee safety conditions and boosting overall efficiency.
- Consumer-focused manufacturing – being able to respond quickly to changes in the market demand.
Watch The Webinar ‘Building Less Wasteful, More Sustainable Production‘.
The Key To Success With AI And Machine Learning For Manufacturing
Certainly, it’s impossible to miss the rapid rise of AI technology – both in general and specifically in the context of manufacturing. It seems like every other technology-related article (and practically every single vendor vying for our attention) uses terms like AI, machine learning, digitization, automation, Industry 4.0 and Industrial Artificial Intelligence, almost as a form of punctuation.
As a result, the expectations when it comes to AI are often wildly off-base, varying from an all-encompassing solution to all your business problems to a deep skepticism any time “AI” is even uttered.
Find The Right Use Case
But, as with any technology, the truth is really somewhere in between. AI can be extremely effective – in the right context. Understanding those contexts, and the kinds of AI technology that apply to them, is the key to setting realistic business goals for AI adoption.
Artificial Intelligence is not a silver bullet. No solution will solve all, or most, of your problems. As a rule of thumb, AI works best when it is applied to solving a specific problem, or a very closely-related set of problems. “General AI” is something to be wary of: if a vendor claims to do everything, then they probably do nothing particularly well.
Which brings us back to the topic of AI in manufacturing. There are numerous potential applications for AI and Machine Learning in manufacturing, and each use case requires a unique type of Artificial Intelligence.
In the guide below, we present a simple, effective formula to select the right Industrial AI solution to address your specific manufacturing challenges and goals – focusing specifically on Machine Learning AI, since that is where the most exciting and impactful innovations are occurring.
The formula can be encapsulated in a simple diagram and methodology called “The Industrial AI Quadrant”.
Next-Generation Optimization For Manufacturers With Machine Learning
The two major use cases of Machine Learning in manufacturing are Predictive Quality & Yield, and Predictive Maintenance.
Only Do Maintenance When It’s Needed
Predictive Maintenance is the more commonly known of the two, given the significant costs maintenance issues and associated problems can incur, which is why it is now a fairly common goal amongst manufacturers.
Instead of performing maintenance according to a predetermined schedule, or using SCADA systems set up with human-coded thresholds, alert rules and configurations, predictive maintenance uses algorithms to predict the next failure of a component/machine/system. Personal can then be alerted to perform focused maintenance procedures to prevent the failure, but not too early so as to waste downtime unnecessarily.
By contrast, traditional manual and semi-manual approaches don’t take into account the more complex dynamic behavioral patterns of the machinery, or the contextual data relating to the manufacturing process at large. For example, a sensor on a production machine may pick up a sudden rise in temperature. A static rule-based system would not take into account the fact that the machine is undergoing sterilization, and would proceed to trigger a false-positive alert.
The advantages are numerous and can significantly reduce costs while eliminating the need for planned downtime in many cases.
By preempting a failure with a machine learning algorithm, systems can continue to function without unnecessary interruptions. When maintenance is needed, it’s very focused – technicians are informed of the components that need inspection, repair and replacement; which tools to use, and which methods to follow.
Predictive maintenance also leads to a longer Remaining Useful Life (RUL) of machinery and equipment since secondary damage is prevented while smaller labour forces are needed to perform maintenance procedures.
Find The Hidden Causes Of Losses
Predictive Quality and Yield – sometimes referred to as just “Predictive Quality” – is a more advanced use case of Industrial Artificial Intelligence, that reveals the hidden causes of many of the perennial process-based production losses manufacturers face on a daily basis. Examples include quality, yield, waste, throughput, energy efficiency, emissions, and more – essentially any loss caused by process inefficiencies.
Predictive Quality and Yield automatically identifies the root causes of process-driven production losses using continuous, multivariate analysis, powered by Machine Learning algorithms that are uniquely trained to intimately understand each individual production process.
Automated recommendations and alerts can then be generated to inform production teams and process engineers of an imminent problem, and seamlessly share important knowledge on how to prevent the losses before they happen.
Reducing these types of losses has always been a struggle for manufacturers of all stripes. But in today’s marketplace, this mission is more important than ever before.
On the one hand, consumers’ expectations are higher than ever before; global consumer habits are gradually “westernizing”, even as the population boom continues. According to numerous surveys, the global population will grow by 25% by 2050, equating to some 200,000 additional mouths to feed every day. On the other hand, consumers have never had so many alternatives available to them, in almost every product imaginable. Recent surveys indicate that this wealth of options means consumers are increasingly likely to permanently ditch even their favorite brands if, for example, a product isn’t available on the shelf.
Against such a backdrop, manufacturers can no longer afford to take process inefficiencies, and their associated losses, in their stride. Every loss in terms of waste, yield, quality or throughput chips away at their bottom line and hands another inch to the competition.
The challenge for many manufacturers is that they eventually hit a glass ceiling in terms of process optimization. Some inefficiencies don’t have any obvious cause, and process experts are left at a loss to explain them. That’s where Machine Learning – and particularly Automated Root Cause Analysis – can really save the day.
Two Main Categories Of Machine Learning – And How They Relate To Manufacturing
Machine Learning can be split into two main techniques: Supervised and Unsupervised.
1) Supervised Machine Learning
In manufacturing use cases, supervised machine learning is the most commonly used technique since it leads to a predefined target: we have the input data; we have the output data; and we’re looking to map the function that connects the two variables.
Supervised machine learning demands a high level of involvement – data input, data training, defining and choosing algorithms, data visualizations, and so on. The goal is to construct a mapping function with a level of accuracy that allows us to predict outputs when new input data is entered into the system.
Initially, the algorithm is fed from a training dataset, and by working through iterations, continues to improve its performance as it aims to reach the defined output. The learning process is completed when the algorithm reaches an acceptable level of accuracy.
In manufacturing, there are two most common Supervised Learning approaches:
Regression And Classification
These two approaches share the same goal: to map a relationship between the input data (from the manufacturing process) and the output data (known possible results such as quality or waste losses, part failure, overheating etc.)
Regression
Regression is used when data exists within a range (eg. temperature, weight), which is often the case when dealing with data collected from sensors.
In manufacturing, regression can be used to calculate an estimate for the Remaining Useful Life (RUL) of an asset. This is a prediction of how many days or cycles we have before the next component/machine/system failure.
For regression, the most commonly used machine learning algorithm is Linear Regression, being fairly quick and simple to implement, with output that is easy to interpret. An example of linear regression would be a system that predicts temperature, since temperature is a continuous value with an estimate that would be simple to train.
Classification
When data exists in well-defined categories, Classification can be used. An example of Classification that we’re all familiar with is the email filter algorithm that decides whether an email should be sent to our spam folder, or not. Classification is limited to a boolean value response, but can be very useful since only a small amount of data is needed to achieve a high level of accuracy.
In machine learning, common Classification algorithms include naive Bayes, logistic regression, support vector machines and Artificial Neural Networks.
2) Unsupervised Machine Learning
With Supervised machine learning we start off by working from an expected outcome and train the algorithm accordingly. Unsupervised learning is suitable for cases where the outcome is not yet known.
Clustering
In some cases, not only will the outcome be unknown to us, but information describing the data will also be lacking (data labels). By creating clusters of input data points that share certain attributes, a Machine Learning algorithm can discover underlying patterns.
Clustering can also be used to reduce noise (irrelevant parameters within the data) when dealing with extremely large numbers of variables.
Artificial Neural Networks
In the manufacturing sector, Artificial Neural Networks are proving to be an extremely effective Unsupervised learning tool for a variety of applications including production process simulation and Predictive Quality Analytics.
The basic structure of the Artificial Neural Network is loosely based upon how the human brain processes information using its network of around 100 billion neurons, allowing for extremely complex and versatile problem solving.
This ability to process a large number of parameters through multiple layers makes Artificial Neural Networks very suitable for the variable-rich and constantly changing processes common to manufacturing. Moreover, once properly trained, an Artificial Neural Network can demonstrate a high level of accuracy when creating predictions regarding the mechanical properties of processed products, enabling cuts in the cost of raw materials.
Data Preparation: Garbage In, Garbage Out
Machine learning is all about data, so understanding some key elements about the quality and type of data needed is extremely important in ensuring accurate results.
With Predictive Quality and Yield, for example, we’re focused on process inefficiencies. Therefore, it makes sense to start by collecting historical data about the performance of the line or lines in question, as well as the losses incurred over time, in order to form predictions about future potential losses.
To get the fullest, most accurate picture possible, that data should be gathered from as many sources as possible, since manufacturing processes – especially more complex ones – are affected by a very wide range of factors that are often interdependent. This can include everything from process data, quality data, raw materials, and even external factors like weather and temperature.
Next, and just as importantly, we need to decide what question we want the Machine Learning model to answer – and whether it is possible to answer this question using the data that’s available.
After all, to get the most out of an Industrial Artificial Intelligence/Machine Learning solution, manufacturers need to know which AI solution is best suited for their own unique sets of challenges.
Read The White Paper ‘The Power of Augury’s AI’. Or to learn more about how our AI is making the jobs of manufacturers easier, get in touch today.